Essential Tools for Data Analysis in Agriculture
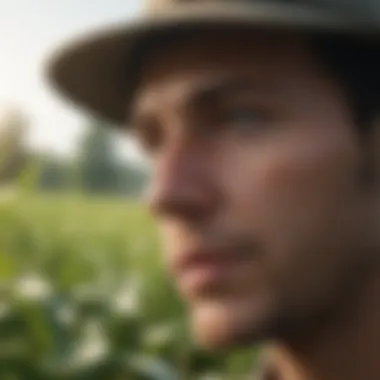
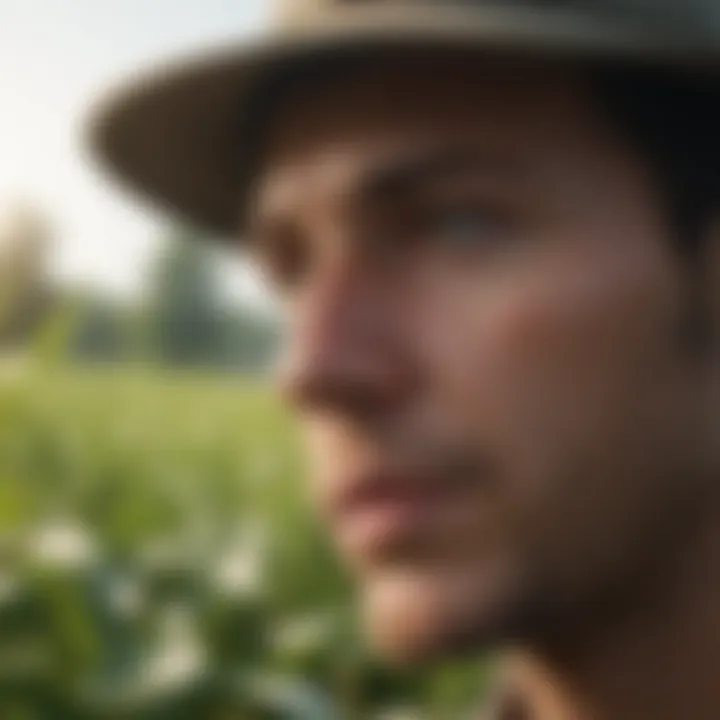
Intro
In the ever-evolving world of agriculture, the role of data analysis cannot be overstated. Agricultural research has grown to incorporate various sophisticated methodologies and tools, allowing for a deeper understanding of farming practices, crop yields, and sustainable techniques. As farmers and researchers alike strive for optimal production and environmental balance, it becomes vital to adopt tools that enhance efficiency and accuracy. This section opens the door to exploring current trends, essential techniques, and the technology shaping the future of farming today.
Current Trends in Agriculture and Horticulture
Overview of Recent Innovations
Agriculture today is on the cutting edge, with innovations like precision farming leading the charge. Farmers are increasingly relying on satellite imagery and IoT devices to monitor crop health in real-time. These technologies generate vast amounts of data, necessitating robust analysis to convert these insights into actionable strategies. For instance, by using drones, researchers can gather detailed aerial views of fields, pinpointing areas needing attention without the labor of manual inspections. The shift towards data-driven decision-making heralds a new era in farming practices.
Sustainable Practices Gaining Popularity
Sustainability has become more than just a buzzword; itâs at the heart of modern agricultural practices. Methods like regenerative agriculture are becoming the talk of the town, focusing on soil health and ecosystem balance. Farmers are now using cover crops and organic composting to improve soil quality and fertility. This holistic view often intersects with data analysis, where assessing soil nutrient levels through data points allows for tailored interventions.
"The use of data in agriculture is not just about increasing yields; it's about creating healthier ecosystems and long-term viability."
Essential Farming Techniques
Soil Health Management
Understanding soil health is foundational in agriculture. The use of data analysis tools like soil sensors helps in determining moisture levels, pH, and nutrient availability. These tools enable farmers to make more informed decisions about irrigation and fertilization. For example, a farmer utilizing a soil moisture sensor can identify drought conditions early, enabling them to adjust their watering schedules effectively, thus conserving resources.
Pest and Disease Control Strategies
Predictive analytics is another key area in agricultural research. By analyzing data on weather patterns and pest populations, farmers can anticipate disease outbreaks before they occur. Software that integrates historical disease data with current climatic conditions allows for targeted interventions, reducing chemical applications and promoting ecological balance.
Tools and Technology in Farming
Overview of Advanced Farming Tools
The technological landscape in agriculture is rich with tools aimed at enhancing productivity. Some notable technologies include:
- GIS (Geographic Information Systems): Enables mapping and analyzing spatial data to make informed land use decisions.
- Farm Management Software: Supports farmers in tracking finances, crop rotations, and compliance. For instance, tools like Ag Leader and Trimble offer comprehensive solutions for managing multiple aspects of farming.
- Autonomous Vehicles: Tractors and harvesters equipped with AI are automating planting and harvesting tasks, thus increasing efficiency.
Case Studies on the Use of Technology
To illustrate the impact of these tools, consider the case of a local farmer using GIS software to manage a 200-acre farm. By analyzing soil variability, they were able to implement variable-rate planting, resulting in a significant yield increase while reducing input costs. Another example is a cooperative that adopted drone technology for disease detection. This not only saved time but also led to more precise pesticide applications, which in return minimized environmental impact.
Prologue to Data Analysis in Agriculture
Data analysis in agriculture is not merely an accessory; itâs the backbone supporting decision-making in this pivotal field. The agricultural sector is increasingly driven by data, and as a result, the ability to analyze this data holds tremendous significance. Farmers and researchers are not just weighing their options based on gut feelings anymore. Instead, they rely on solid data to make choices that can impact yield, sustainability, and profitability.
Importance of Data Analysis
The importance of data analysis in the agricultural realm canât be overstated. With technology advancing at breakneck speed, gathering data is easier than it has ever been before. Weather patterns, soil health, and crop performance can all be monitored through various means, be it sensors or satellite imagery. But collecting this data is just half the battle. What good is a treasure trove of information if it doesnât lead to actionable insights?
Here are a couple of reasons why data analysis in agriculture is crucial:
- Informed Decision-Making: By analyzing data, farmers can make decisions that are based on facts rather than speculation. This leads to optimized resource use and better crop yields.
- Risk Management: Data helps farmers anticipate problems and mitigate risks. Whether itâs a chance of drought or pest infestations, knowing the data patterns can guide farmers to act before itâs too late.
"Data-driven decisions in agriculture can mean the difference between thriving and merely surviving."
Overview of Common Data Analysis Tools
An array of tools is at the disposal of modern agricultural researchers and practitioners. Understanding which tools to use based on the specific data involved is imperative. Broadly speaking, data analysis tools can be categorized into several types that cater to different facets of agricultural operations.
- Statistical Software: Programs like R and Python offer extensive libraries for statistical analysis, making them indispensable when dealing with complex datasets.
- Visualization Tools: Tools like Tableau and Power BI enable users to transform numbers into visual stories. As the saying goes, a picture is worth a thousand words, and this has never been truer for data analysis.
- Machine Learning Software: Advancements in machine learning have opened new frontiers in predicting agricultural trends, allowing for data-driven planning that can optimize yield and reduce waste.
In this article, weâre going to delve deeper into these tools and methodologies, illuminating how they can enhance the agricultural research landscape and contribute to sustainable practices.
Types of Data in Agricultural Research
In agricultural research, understanding the various types of data is crucial for informed decision-making. The categorization of data into quantitative and qualitative types shapes the methods and tools used in the analysis, contributing to the accuracy and relevance of findings. By grasping these distinctions, researchers can better tailor their strategies to meet specific research aims. For instance, quantitative data brings in measurable formatsânumbers, statistics, and scoresâthat can be analyzed mathematically. Conversely, qualitative data provides insights that are more subjective, involving feelings, opinions, and observations that extend beyond mere numbers.
This duality in data types shines a light on the broader landscape of agricultural research, where each type plays its role in driving knowledge forward. Balancing the two allows researchers to develop a more holistic understanding of the agricultural sector, leading to decisions that are both well-informed and empathetic to the various factors at play in farming practices.
Quantitative vs. Qualitative Data
Quantitative data refers to any data that can be expressed numerically, such as crop yields, soil pH levels, or rainfall measures. This type of data is essential because it quantifies relationships, enables statistical analysis, and supports predictive modeling. For researchers in agriculture, being able to measure these variables effectively contributes to the objective assessment of farming practices and outputs. Tools such as spreadsheets or advanced statistical software like R or Python become invaluable for processing this data effectively.
Qualitative data, on the other hand, deals with non-numeric information that can provide context to the numbers. This includes interviews, focus groups, and local knowledge about crop varieties or pest management techniques. To ignore qualitative insights means potentially overlooking critical local wisdom that carriers of traditional agricultural practices could contribute. It brings depth to the analysis, enhancing the understanding of farmersâ challenges and practices beyond what quantitative data can show. This approach is crucial, particularly in developing agricultural strategies that are sensitive to the needs of diverse farming communities.
Field Data Collection Methods
Field data collection is the backbone of agricultural research, allowing for real-world application of theories and methodologies. Understanding how to gather data from the field is essential for accurate and reliable research outcomes. Here are some popular methods used:
Surveys
Surveys are a classic method for gathering information from farmers or agricultural stakeholders. They enable researchers to collect information on a broad scale, asking specific questions that can be statistically analyzed later. A key characteristic of surveys is their ability to gather a large amount of data in a structured mannerâmaking them a beneficial choice in agricultural research for both qualitative and quantitative data needs.
The unique feature of surveys lies in their flexibility; they can be conducted in person, over the phone, or even through digital platforms. Advantages here include reaching a diverse audience across geographical boundaries, but itâs important to note that poorly designed surveys can lead to bias and inaccuracies in results.
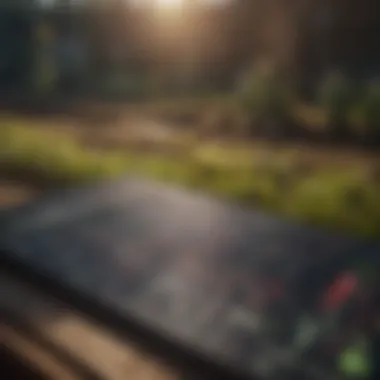
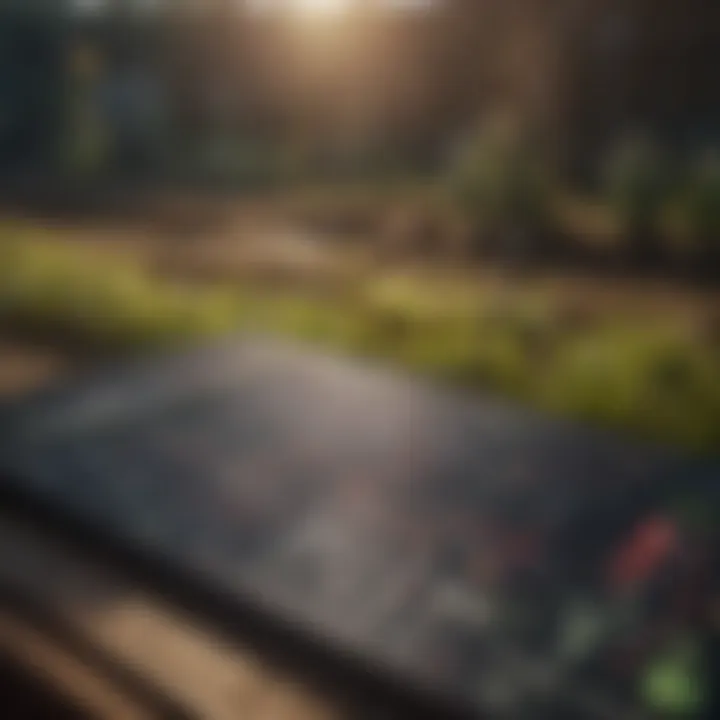
Remote Sensing
Remote sensing involves collecting data through satellite or aerial imagery, allowing researchers to assess large agricultural areas without the need to be on-site. This method's key characteristic is its capacity for providing real-time data on crop health, land use, and environmental factors, which are hugely beneficial for precision agriculture.
The unique feature of remote sensing is its ability to gather extensive data across vast areas quickly. However, interpreting the data can sometimes require advanced training or technical expertise, posing a challenge for researchers unfamiliar with this technology.
Ground Truthing
Ground truthing complements remote sensing by validating data collected through remote means with on-the-ground evidence. This method is crucial, as it ensures the accuracy of the remote data analysis. A key characteristic of ground truthing is its confirmatory nature, as it allows researchers to match real-world conditions with satellite data.
This method shines with its unique ability to populate the data with qualitative insights, such as local biodiversity or the presence of pests. Though time-consuming and resource-intensive, ground truthing brings about the highest level of confidence in data analysis, validating the broader insights gleaned from technology-driven methods.
"Understanding different types of data and effective methods for collection is fundamental to achieving successful outcomes in agricultural research."
Ultimately, the integration of these data types and collection methods enriches agricultural research, leading to insights that could drive sustainable practices and innovations.
Data Management Tools
Data management tools play a crucial role in the sphere of agricultural research. They not only enable seamless organization of data but also ensure that data integrity, quality, and security are maintained throughout the research process. This section systematically explores the importance of effective data management, examines database management systems, and discusses various data storage solutions which can facilitate better data handling.
Importance of Data Management
In agricultural research, data can be a double-edged sword. On one side, when managed effectively, it can provide valuable insights that lead to improved farming practices; on the other hand, poor data management can lead to misinterpretations and potentially disastrous consequences. The significance of data management includes:
- Streamlining Data Collection: Efficient management allows researchers to plan their data collection strategies better.
- Enhancing Data Quality: By establishing protocols for data entry and maintenance, researchers can minimize errors.
- Facilitating Data Sharing: Good data management practices ease collaboration among agricultural scientists, which is vital for interdisciplinary research.
Ultimately, solid data management helps transform raw data into actionable knowledge, setting the stage for informed decisions in agriculture.
Database Management Systems
Database Management Systems (DBMS) are essential for organizing agricultural data. They provide the structural capability to store, retrieve, and manage data efficiently. We can break these systems down into two primary categories:
SQL Databases
SQL databases are a type of relational database known for their robust structure. They work well with structured data, making them a beloved choice among researchers for their capability to handle substantial datasets.
- Key Characteristic: The use of Structured Query Language (SQL) to manage and manipulate data.
- Popular Choice: SQL databases like PostgreSQL and MySQL are noted for their reliability and ease of use, especially for users who expect data relationships to be defined clearly.
A unique feature of SQL databases is their ability to enforce data integrity through constraints and rules, ensuring that inaccurate data doesnât sneak into the analysis phase. While they shine in relational scenarios, they can sometimes struggle with unstructured or semi-structured data, limiting their versatility in certain agricultural research contexts.
NoSQL Databases
NoSQL databases offer a different flavor, featuring a more flexible schema design. They are particularly suitable for handling a diverse range of data types, such as text, images, or even sensor data from equipment used in agriculture.
- Key Characteristic: The ability to scale horizontally and manage vast amounts of unstructured data efficiently.
- Beneficial Choice: Options like MongoDB and Cassandra are becoming increasingly popular for their speed and scalability, making them ideal for agricultural research that gathers data from various IoT devices.
One standout feature of NoSQL databases is their support for real-time data access, allowing researchers to perform live analysis of incoming data streams. However, the downside can be the compromise on data consistency, which might be an issue for projects needing stringent data validation.
Data Storage Solutions
Data storage solutions form the backbone of data management in agricultural research. They can be broadly categorized into cloud storage and local storage options, each with its own strengths and weaknesses.
Cloud Storage
Cloud storage solutions have gained traction in various fields, including agriculture, due to their inherent advantages over traditional storage methods.
- Key Characteristic: Accessibility from any device with an internet connection, promoting collaboration.
- Popular Choice: Services like Google Drive and Dropbox are often favored for their ease of use and scalability.
A unique benefit of cloud storage is the automation of backups and updates, which alleviate the burden of data loss that can occur with local storage. A potential drawback, however, lies in data security concerns, as sensitive information could be vulnerable to breaches if appropriate measures arenât taken.
Local Storage Options
Local storage refers to traditional methods, where data is stored directly on the user's physical devices, such as hard drives or local servers.
- Key Characteristic: Full control over one's data, including security.
- Beneficial Choice: Many researchers are inclined toward external hard drives or dedicated server setups due to the direct access they provide without the need for internet connectivity.
A notable feature of local storage is that it can offer higher data retrieval speeds, particularly when dealing with large datasets in a controlled environment. Nevertheless, this comes with limited scalability and the risk of data loss if physical devices fail, underscoring the importance of regular backups.
Understanding these data management tools is pivotal for fostering smooth and effective research processes in agriculture, ultimately leading to improved outcomes and insights.
Statistical Analysis Software
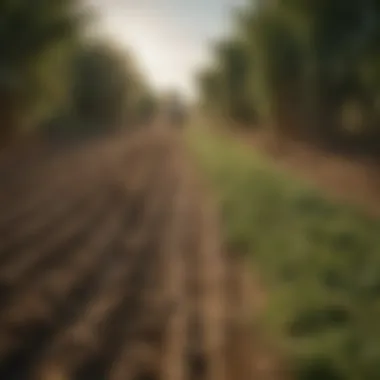
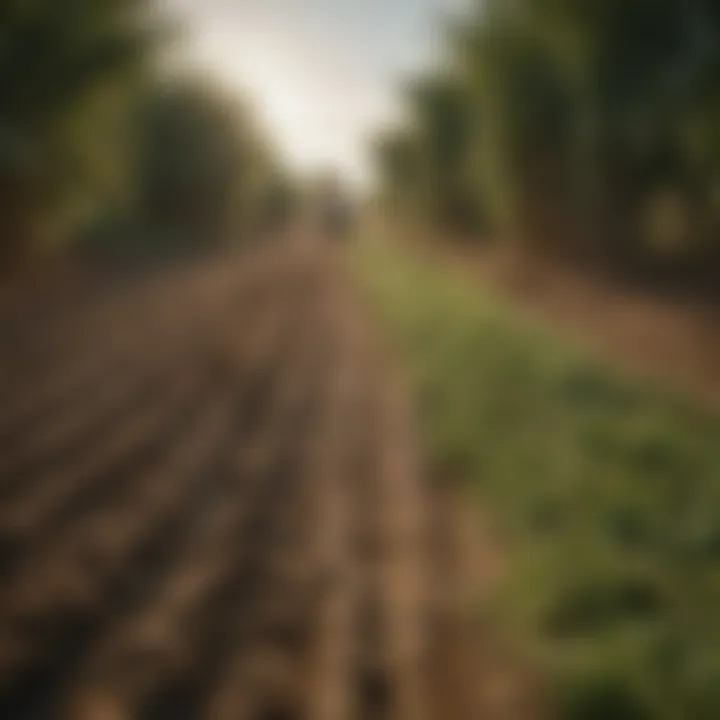
Statistical analysis software serves as a backbone for data processing in agricultural research. With vast amounts of data generated from various sources, effective analysis is paramount. Researchers need tools that not only assist in managing these datasets but also help draw meaningful conclusions that can influence agricultural practices and policies. The importance of statistical software cannot be overstated, especially when working with complex data sets that involve diverse variables typical in agricultural studies.
Prelude to Statistical Software
Statistical software provides a platform where researchers can manipulate, model, and visualize data efficiently. It simplifies intricate calculations, allowing researchers to focus on interpretation rather than on the mechanics of data analysis. This software offers capabilities like hypothesis testing, regression analysis, and various advanced statistical techniques that provide insights into environmental factors affecting agricultural yield. Using these tools enables researchers to unearth patterns that might not be evident at first glance, making it invaluable in the agricultural landscape.
R Programming for Data Analysis
Basics of R
R has carved a niche for itself in the realm of statistical computing due to its extensive package ecosystem. Its strength lies in its flexibility and the ability to handle a multitude of data types. Researchers can implement R in various aspects of data analysisâfrom basic descriptive statistics to complex predictive modeling.
A key characteristic of using R is its open-source nature, allowing users to access and modify its code. This fosters a collaborative community that constantly develops new packages to extend the softwareâs functionality. One notable feature of R is the diverse plotting options available, which can visually represent data findings in a more graspable format. While R is powerful, it's worth noting that its steeper learning curve might be a barrier for some users initially, but the knowledge gained is often rewarding.
Advanced Statistical Functions
Advanced statistical functions in R elevate the analysis capabilities to another level. For instance, functions such as for linear modeling and for generalized linear models streamline the process of fitting models to data. Such functionality is particularly valuable when working with agricultural data that often necessitates sophisticated models to predict outcomes based on several predictors.
This feature of R allows researchers to apply state-of-the-art statistical techniques while also facilitating replication of studies by providing clear and reproducible analysis. However, with great power comes some level of complexity, meaning additional effort is needed to master these functions. Those committed to learning will certainly find that R serves their analytical needs abundantly well.
Python for Data Science
Data Manipulation with Pandas
Pandas has become synonymous with data manipulation in the Python ecosystem. It excels at handling time series, labeled data, and allows for easy cleaning and transforming in preparation for analysis. This efficiency is crucial in agriculture where data often comes from disparate sources, thus requiring proper alignment and integration.
The hallmark characteristic that sets Pandas apart is its straightforward DataFrame structure, which offers functionalities similar to SQL and Excel combined. This is a tremendous asset to researchers who may find themselves less familiar with codingâproviding a familiar tabular data structure. However, despite its user-friendly interface, the initial setup and learning syntax can take some time, which may discourage some users.
Statistical Methods with SciPy
SciPy is another gem in the Python library for those who delve into more rigorous statistical analysis. With features for optimization, integration, interpolation, eigenvalue problems, and others, it provides comprehensive tools for anyone looking to perform detailed scientific and technical computations. Researchers can employ statistical tests like t-tests, chi-square tests, and more without needing to rely on external tools.
A significant advantage of SciPy is its seamless integration with NumPy and other libraries, offering a cohesive experience for users. Its comprehensive documentation also caters to both novice and advanced users, providing guidance in a straightforward manner. However, similar to other powerful tools, it requires a reasonable level of familiarity with programming concepts to fully harness its potential.
Data Visualization Tools
In the realm of agricultural research, data visualization tools play a crucial role in transforming raw data into comprehensible visuals that tell a story. These tools enable researchers and farmers alike to glean insights quickly, making complex data sets more accessible and actionable. When dealing with vast amounts of data, the ability to visualize that information can mean the difference between overwhelming confusion and clear understanding. This section delves into the essential aspects of data visualization tools, highlighting their benefits and considerations for use.
Role of Visualization in Data Analysis
Data visualization acts as a bridge between analytical results and decision-making. The essence of visual representation is that it simplifies data analysis, allowing for immediate recognition of patterns, trends, and outliers. Visual aids can highlight important relationships and disparities that would otherwise be hidden in raw statistics. For agricultural researchers, this means being able to spot, say, which fertilizers lead to higher yields across different crops quickly.
Moreover, visuals often facilitate better communication of findings. Stakeholders range from farmers to policymakers, and each might come with varying levels of statistical literacy. An intuitive chart can convey messages that text-filled reports might fail to deliver. Thus, harnessing the power of visualization not only aids in analysis but also in advocacy for data-driven agricultural practices.
Popular Visualization Software
Tableau
Tableau stands out in the field of data visualization due to its user-friendly interface and powerful capabilities. One specific aspect that makes Tableau remarkable is its ability to handle large volumes of data from multiple sources, integrating seamlessly and providing insight at various levels of detail.
A key characteristic of Tableau is its emphasis on interactivity. Users can create dynamic dashboards that encourage exploration of the dataset. This interactivity is especially beneficial in agriculture, where conditions can quickly change, and real-time data analysis becomes essential. One unique feature of Tableau is its "Show Me" functionality, which suggests the best type of visualization for a given dataset, guiding usersâregardless of expertiseâtoward effective presentations. The downside? Tableau can be costly, which might deter smaller farms or research projects from utilizing its full potential.
Power BI
Power BI emerges as a strong contender, particularly for those already embedded within the Microsoft ecosystem. Its integration with Excel and other Microsoft products is a specific aspect that simplifies data manipulation and presentation. Users can leverage familiar tools while expanding their analytical capabilities.
A key characteristic that defines Power BI is its focus on collaborative work. Teams can share insights and dashboards effortlessly, breaking down silos within agricultural research organizations. One notable feature is its cloud-based service, enabling remote access and real-time collaboration. However, a common critique is that its user interface may come with a learning curveâespecially for individuals not used to Microsoft's suite of applications.
Matplotlib and Seaborn
Python offers rich libraries for visualization, with Matplotlib and Seaborn standing at the forefront. A notable specific aspect of these tools is their extensibility; both libraries allow users a high degree of customization, making them perfect for nuanced agricultural data representation.
Matplotlib is foundational, providing various plotting capabilities. It is complemented by Seaborn, which builds on Matplotlib's capabilities by offering aesthetically pleasing defaults and additional features for statistical plotting. The primary advantage of using these libraries is their flexibilityâthey cater well to both simple graphs and intricate data visualizations. However, they do demand some programming knowledge, which can be a barrier for users who do not code.
Machine Learning Applications in Agriculture
Machine learning has been making waves in numerous fields, and agriculture is no exception. Its applications can streamline processes, enhance decision-making, and ultimately lead to higher yields and lower costs. In the quest for sustainability, understanding how machine learning functions in agriculture allows farmers and researchers to tackle challenges that in the past seemed insurmountable. From predicting crop diseases to optimizing irrigation systems, the potential benefits are extensive. Employees of farms can leverage these advanced analytical methods to make informed choices based on vast amounts of data that would otherwise be overwhelming.
Prolusion to Machine Learning
Machine learning is a subset of artificial intelligence that enables computers to learn from, and make predictions based on data. In agricultural research, this translates to creating models that can forecast outcomes, identify trends, and automate certain processes. The crux of machine learning lies in its ability to improve over time as more data becomes available. For farmers and researchers, harnessing this technology means spending less time crunching numbers and more time applying insights in practical, beneficial ways.
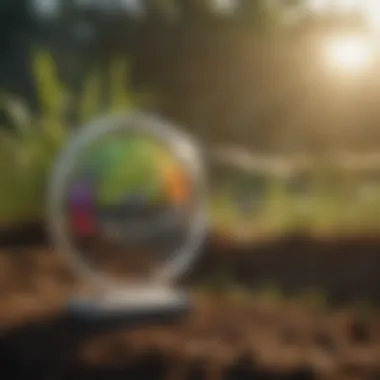
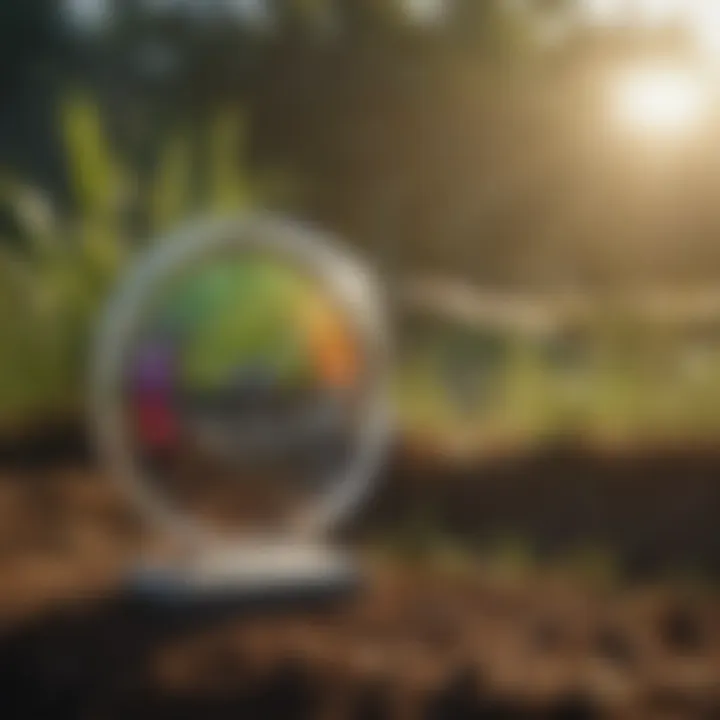
Common Algorithms Used
Supervised Learning
Supervised learning is all about training the model on existing data with clear outcomes, allowing it to predict results for new, unseen data. This approach is a popular choice in agriculture because it can be used for a variety of tasks, such as crop yield prediction or disease detection. A notable characteristic is that it uses labeled examples to learn. For instance, if you have historical data on certain crops along with their yields, the model can learn the relationships and make predictions for the future. The main advantage here is accuracy, but it does have a downside; it requires a substantial amount of labeled data, which can sometimes be hard to come by.
Unsupervised Learning
In contrast to supervised learning, unsupervised learning deals with data that doesnât have labels. It's particularly useful in uncovering hidden patterns and relationships within datasets, making it a valuable tool in agriculture where identifying customer segments or soil types can lead to better-targeted planting strategies. The distinctive feature of this method is its capacity to discover structure in data without prior training. One of the strong suits of unsupervised learning is its flexibility, although a significant challenge is interpreting the results, as there isnât a defined outcome to guide analysis.
Reinforcement Learning
Reinforcement learning operates differently by encouraging algorithms to learn by trial and error, similar to how humans learn new skills. In agriculture, this method can be applied to areas like autonomous farming equipment, where machines adapt their strategies based on various environmental conditions. The key characteristic is feedback. Unlike other models, reinforcement learning relies on the sequences of actions taken, where each decision influences future actions. While it offers adaptability, the downside is that it typically requires extensive computational resources and a significant amount of time to train.
Practical Examples of Machine Learning in Agriculture
Practical applications of machine learning in agriculture are not just theoretical; they can lead to tangible improvements. Here are a few noteworthy examples:
- Crop Disease Prediction: By analyzing historical weather data and plant health information, machine learning models can help predict outbreaks of crop diseases, allowing farmers to act swiftly.
- Yield Optimization: Algorithms can process soil health data along with weather forecasts to recommend the optimal planting strategies and fertilization schedules.
- Resource Monitoring: Machine learning helps in monitoring water usage and can suggest efficient irrigation methods or times, thus conserving water and reducing costs.
"Machine learning transforms data into actionable insights, guiding farmers and researchers towards sustainable practices and improved yields."
In summary, machine learning applications in agriculture offer powerful tools to manage complexities intrinsic to farming. This innovative technology is no longer a mystery; rather, itâs becoming an integral part of modern agricultural practices.
Challenges in Data Analysis for Agriculture
In the realm of agricultural research, the journey towards efficient data analysis is often littered with hurdles. This section delves into the challenges that researchers and farmers frequently encounter, highlighting how these issues can impede the progress of agricultural innovations. Understanding these obstacles is vital, not just for addressing current problems but also for paving the way for future developments in the field.
Data Quality Issues
"Garbage in, garbage out" is a mantra that resonates throughout the world of data analysis. The integrity of the data collected directly influences the accuracy of results and subsequent decisions. In agriculture, data quality can be compromised by several factors:
- Incomplete Data: Often, not all fields or sections of data are recorded in surveys or sensor readings. This gaps can lead to skewed insights.
- Inconsistent Measurement Techniques: Different methods of data collection can produce conflicting results. For instance, moisture readings from various soil types using different instruments can cause discrepancies.
- Legitimacy of Data Sources: There's a tendency to rely on unverifiable sources. Data collected through informal channels might miss crucial validations.
- Temporal Relevance: With agriculture, data from last season may not reflect the current growth patterns or market conditions, leading to outdated analyses.
Addressing these quality issues requires rigorous data validation methods and a structured approach to data collection. Without tackling these challenges, the root of agricultural problems may remain buried beneath layers of unreliable data.
Future Trends in Data Analysis for Agriculture
In an era where information drives decisions, understanding future trends in data analysis for agriculture becomes crucial. With the world continuously evolving, agricultural researchers need to keep pace with the methods and tools that can help optimize farming practices. These trends not only provide insights into more effective farming strategies but also facilitate sustainability and efficiency in the agricultural sector.
Emerging Technologies
Emerging technologies are reshaping how data is collected, analyzed, and applied in agriculture. One prominent trend gaining traction is the development of drone technology. Drones can capture real-time data over extensive land, providing farmers with valuable insights regarding crop health and soil conditions at a fraction of the traditional cost. The ability to monitor crops from above enables precision agriculture, where interventions can be targeted rather than blanket-applied.
Moreover, innovations like artificial intelligence and machine learning are playing significant roles in predicting crop yields and optimizing resource use. For instance, models that analyze historical data can offer forecasts based on various scenarios, helping farmers make educated choices about planting times and fertilizer usage.
"Technological advancements in data analysis can transform agricultural practices, making them more efficient and eco-friendly."
Integration of IoT and Data Analysis
The Internet of Things (IoT) is another significant player in the agricultural data landscape. Sensors installed in fields can collect a myriad of data points, such as soil moisture levels, weather patterns, and crop growth metrics. This data can be integrated with analytical tools to generate insights that drive farming decisions.
A connected farm can react instantaneously to environmental conditions. For instance, if soil moisture is low, irrigation systems can automatically activate, ensuring crops receive the necessary water without wasted resources. Positive outcomes include higher efficiency, lower costs, and improved crop yields.
Moreover, the data collected through IoT devices can inform long-term strategies. Understanding patterns over time can help researchers identify which crops thrive under certain conditions or when pest outbreaks might occur, allowing preventative measures to be implemented before the situation escalates.
End and Recommendations
In the realm of agricultural research, the power of data analysis can hardly be overstated. With technology shaping how farmers cultivate and scientists investigate, drawing conclusions rooted in detailed insights has become paramount. The concluding section of this article encapsulates the key elements discussed and provides practical recommendations for those embarking on their own data analysis journeys.
Data analysis is not merely a procedural task; it serves as the backbone of decision-making in agricultural research. The insights gathered from various tools and methodologies are invaluable in enhancing productivity, achieving sustainable practices, and ultimately fostering innovation. Some benefits are tangible, such as increased crop yields or reduced wastage, while others might contribute to long-term environmental sustainability.
Summarizing Key Insights
Through this discourse, we have fleshed out some significant takeaways:
- Understanding Types of Data: Recognizing the nuances between quantitative and qualitative data is foundational. Each type serves a distinct purpose, whether it's gathering numerical statistics for yield predictions or using open-ended survey responses to assess farmer experiences.
- Data Management is Crucial: Efficient organization and storage of data cannot be overlooked. Data management systems like SQL databases enable the collation of crucial research information, preventing data loss and ensuring easy access.
- Statistical Software Utilization: Programs such as R and Python bring analytical power to the table. They can perform complex computations and visualizations that are essential for understanding patterns in agricultural research.
- Embracing Data Visualization: A good visualization tool, whether Tableau or Matplotlib, can mean the difference between overwhelming data interpretation and clear, actionable insights.
- Machine Learning as a Game Changer: As technology evolves, so do the opportunities for employing machine learning algorithms to predict trends and enhance farming practices.
"Data analysis in agriculture is where insight meets practice, unlocking pathways for sustainable development and efficient farming methods."
Selecting the Right Tools for Research
Selecting suitable tools can be daunting, especially with a plethora of options available. Here are some important considerations when making your choice:
- Research Objectives: Clearly defined goals will help in narrowing down options. Are you looking to visualize data, run statistical analyses, or manage large datasets?
- User Skill Level: Consider the technicality of the tools. For example, R provides advanced capabilities but has a steep learning curve. If your team is more comfortable with simpler applications, software like Excel or Tableau may be preferable.
- Budget Constraints: Certain tools may require subscriptions or one-time purchases. Itâs important to assess the financial implications and opt for tools that offer good value for money without breaking the bank.
- Integration Capabilities: The ability to integrate multiple tools can significantly streamline analysis. Check if the selected tool can work with others you already use, ensuring a smoother workflow.
- Support and Resources: Look for tools that come with comprehensive support, whether through documentation, community forums, or customer service. A resource-laden software can save time when faced with problems.
Ultimately, the right tools can significantly enhance your agricultural research capabilities. By making informed decisions based on the points discussed above, researchers can set the stage for clearer insights and improved results in their endeavors.